Improving representation of bulk snowpack density across landscapes using advanced snow depth data
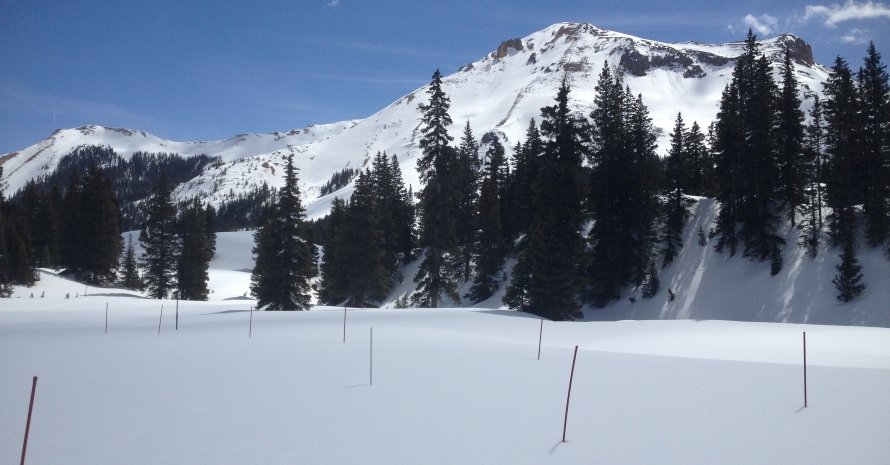
![]() Some technologies for measuring snow depth at different scales |
State of the Science: Snow depth vs. Snow densityAn ongoing technological revolution promises to proliferate availability of snow depth data (see figure left). Approaches with LiDAR and photogrammetry (via drones or time-lapse cameras) can offer unprecedented information about spatial variability of snow depth at a range of scales/extents. Snow depth variability in time can also be monitored at local scales with networks of low-cost ultrasonic sensors and GPS stations. Technological advances have even come to the most traditional method for measuring snow depth (manual probing), through self-logging, GPS-linked snow probes that extend measurement capabilities. In contrast to snow depth measurement, there has been less progress in technologies for snow density measurement. We therefore rely on snowpack models to estimate density variations in space and time. There can be considerable uncertainty sources within and between models, and in many environments, the uncertainty in density estimation is now the chief source of SWE uncertainty. Field studies have shown that snow density exhibits variability in space (albeit more conservatively than snow depth), but the linkages to landscape processes is unclear. This research is motivated to improve the science of snow density estimation in models and to assess how snow density varies across landscapes. |